Quickstart#
AutoEmulate
’s goal is to make it easy to create an emulator for your simulation. Here’s the basic workflow:
import numpy as np
import random
import torch
from autoemulate.compare import AutoEmulate
from autoemulate.experimental_design import LatinHypercube
from autoemulate.simulations.projectile import simulate_projectile
/opt/hostedtoolcache/Python/3.11.11/x64/lib/python3.11/site-packages/autoemulate/compare.py:8: TqdmExperimentalWarning: Using `tqdm.autonotebook.tqdm` in notebook mode. Use `tqdm.tqdm` instead to force console mode (e.g. in jupyter console)
from tqdm.autonotebook import tqdm
seed = 43
np.random.seed(seed)
random.seed(seed)
torch.manual_seed(seed)
<torch._C.Generator at 0x7fe4e8998f10>
Design of Experiments#
Before we build an emulator or surrogate model, we need to get a set of input/output pairs from the simulation. This is called the Design of Experiments (DoE) and is currently not a key part of AutoEmulate
, as this step is tricky to automate and will run on more complex compute infrastructure for expensive simulations. There are lots of sampling techniques, but here we are using Latin Hypercube Sampling.
Below, simulate_projectile
is a simulation for a projectil motion with drag (see here for details). It takes two inputs, the drag coefficient (on a log scale) and the velocity and outputs the distance the projectile travelled. We sample 100 sets of inputs X
using a Latin Hypercube Sampler and run the simulator for those inputs to get the outputs y
.
# sample from a simulation
lhd = LatinHypercube([(-5., 1.), (0., 1000.)]) # (upper, lower) bounds for each parameter
X = lhd.sample(100)
y = np.array([simulate_projectile(x) for x in X])
X.shape, y.shape
((100, 2), (100,))
Comparing emulators#
This is the core of AutoEmulate
. With a set of inputs / outputs, we can run a full machine learning pipeline, including data processing, model fitting, model selection and potentially hyperparameter optimisation in just a few lines of code. First, we initialise an AutoEmulate
object. Then, we run setup(X, y)
, providing the simulation inputs and outputs. Lastly, compare()
will fit a range of different models to the data and evaluate them using cross-validation, returning the best emulator.
# compare emulator models
ae = AutoEmulate()
ae.setup(X, y)
ae.compare()
AutoEmulate is set up with the following settings:
Values | |
---|---|
Simulation input shape (X) | (100, 2) |
Simulation output shape (y) | (100,) |
Proportion of data for testing (test_set_size) | 0.2 |
Scale input data (scale) | True |
Scaler (scaler) | StandardScaler |
Do hyperparameter search (param_search) | False |
Reduce dimensionality (reduce_dim) | False |
Cross validator (cross_validator) | KFold |
Parallel jobs (n_jobs) | 1 |
Pipeline(steps=[('scaler', StandardScaler()), ('model', GaussianProcess())])In a Jupyter environment, please rerun this cell to show the HTML representation or trust the notebook.
On GitHub, the HTML representation is unable to render, please try loading this page with nbviewer.org.
Pipeline(steps=[('scaler', StandardScaler()), ('model', GaussianProcess())])
StandardScaler()
GaussianProcess()
We can have a look at the average cross-validation results for each model:
ae.summarise_cv()
model | short | rmse | r2 | |
---|---|---|---|---|
0 | GaussianProcess | gp | 517.275126 | 0.995274 |
1 | RadialBasisFunctions | rbf | 1069.159255 | 0.976833 |
2 | ConditionalNeuralProcess | cnp | 2644.153921 | 0.855675 |
3 | SupportVectorMachines | svm | 3182.071644 | 0.824338 |
4 | RandomForest | rf | 3367.341393 | 0.751152 |
5 | GradientBoosting | gb | 3462.521023 | 0.648642 |
6 | SecondOrderPolynomial | sop | 4127.016303 | 0.606897 |
7 | LightGBM | lgbm | 5478.203883 | 0.292631 |
And create plots comparing the models:
ae.plot_cv()
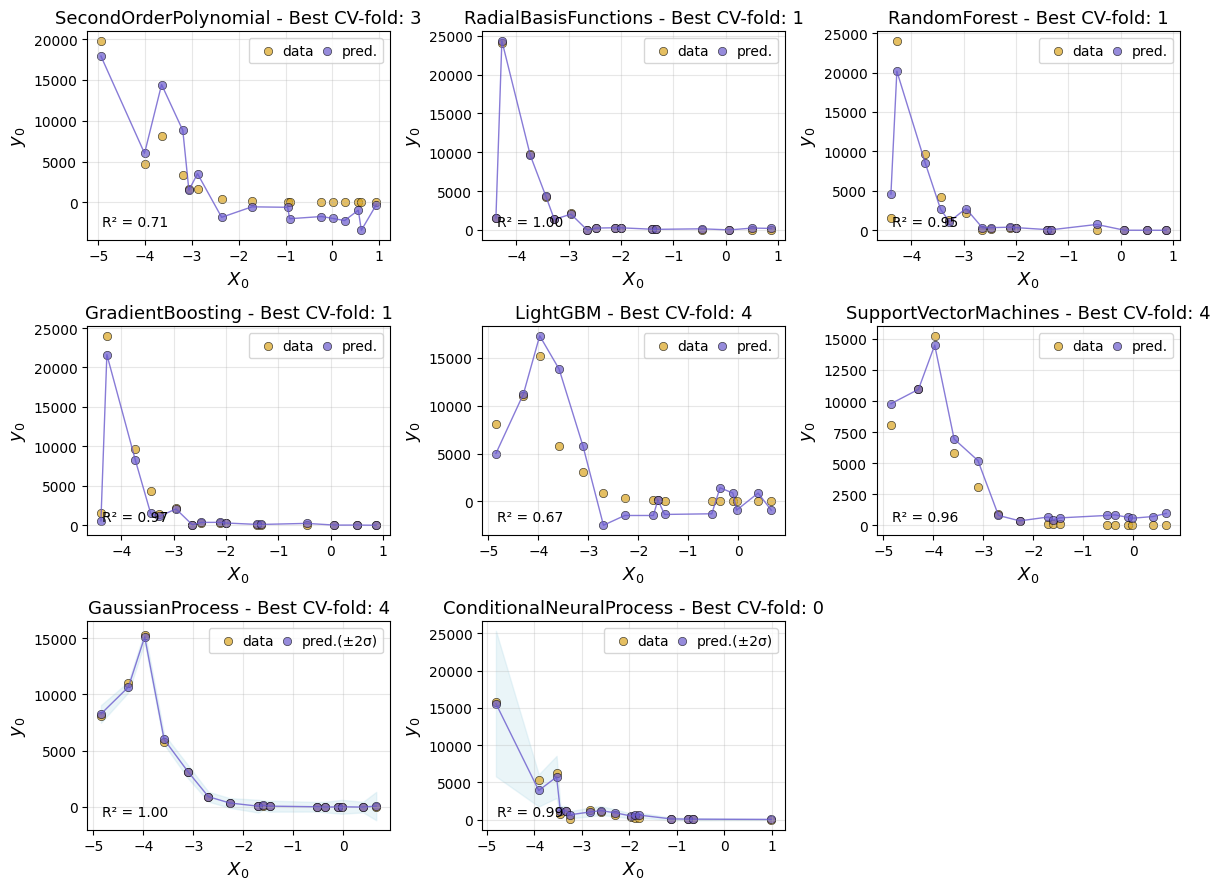
Evaluating on the test set#
AutoEmulate
has already split the data into a training set and a test set. After looking at the cross-validation results, we can retrieve a fitted emulator and evaluate it on the test set. The GP predicts well on unseen data.
gp = ae.get_model("GaussianProcess")
ae.evaluate(gp)
model | short | rmse | r2 | |
---|---|---|---|---|
0 | GaussianProcess | gp | 101.2648 | 0.9997 |
But it’s always useful to plot the predictions too.
ae.plot_eval(gp, input_index=[0, 1])
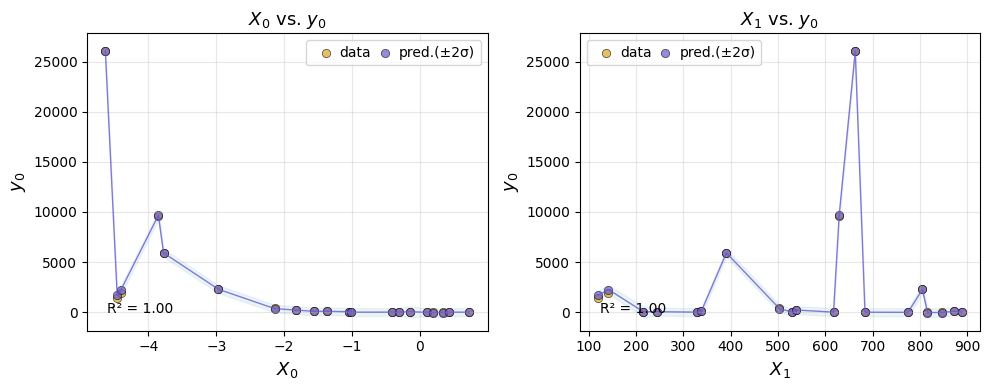
Refitting the emulator#
Before applying the emulator, we refit it on the entire dataset, including training and test set. This is done with the refit()
method.
gp_final = ae.refit(gp)
Predictions#
We can use the best model to make predictions for new inputs. Emulators in AutoEmulate
are scikit-learn
estimators, so we can use the predict
method to make predictions.
gp_final.predict(X[:10])
array([ 5.93070430e+03, 8.09617772e+03, 1.64336424e+04, 8.03823705e+03,
1.02827644e+02, -4.28234121e+00, 6.29678458e+00, 6.02840269e+01,
1.08400982e+04, 6.95614838e+00])
Sensitivity analysis#
A common task for emulators is Global Sensitivity Analysis. We can perform this with the sensitivity_analysis
method.
si = ae.sensitivity_analysis(gp_final)
si
output | parameter | index | value | confidence | |
---|---|---|---|---|---|
0 | y1 | X1 | S1 | 0.923337 | 0.125360 |
1 | y1 | X2 | S1 | 0.390887 | 0.094537 |
0 | y1 | X1 | ST | 0.626819 | 0.145148 |
1 | y1 | X2 | ST | 0.096640 | 0.062260 |
0 | y1 | [X1, X2] | S2 | 0.295638 | 0.344865 |
ae.plot_sensitivity_analysis(si, index="S1", figsize=(3, 3))
---------------------------------------------------------------------------
TypeError Traceback (most recent call last)
Cell In[12], line 1
----> 1 ae.plot_sensitivity_analysis(si, index="S1", figsize=(3, 3))
File /opt/hostedtoolcache/Python/3.11.11/x64/lib/python3.11/site-packages/autoemulate/compare.py:624, in AutoEmulate.plot_sensitivity_analysis(self, results, index, n_cols, figsize)
604 def plot_sensitivity_analysis(self, results, index="S1", n_cols=None, figsize=None):
605 """
606 Plot the sensitivity analysis results.
607
(...) 622
623 """
--> 624 return _plot_sensitivity_analysis(results, index, n_cols, figsize)
File /opt/hostedtoolcache/Python/3.11.11/x64/lib/python3.11/site-packages/autoemulate/sensitivity_analysis.py:274, in _plot_sensitivity_analysis(results, problem, index, n_cols, figsize)
253 """
254 Plot the sensitivity analysis results.
255
(...) 270
271 """
272 with plt.style.context("fast"):
273 # prepare data
--> 274 results = _validate_input(results, problem, index)
275 unique_outputs = results["output"].unique()
276 n_outputs = len(unique_outputs)
TypeError: _validate_input() takes 2 positional arguments but 3 were given
Saving / loading models#
Lastly, we can save and load an emulator. To load, we need an initialised AutoEmulate
object. This will ensure that the environment in which the model was saved is similar to the environment in which it is loaded.
# save & load best emulator
# ae.save(gp_final, "gp_final")
# emulator = ae.load("gp_final")